Through a combination of predictive algorithms, prescriptive optimization-based modeling, and a live operational planning system, artificial intelligence (AI) can enable continuous improvement throughout the forestry supply chain and provide real competitive advantage in fast-changing markets.
Global industries are benefiting from a confluence of improved data acquisition and forest management platforms and advancements in artificial intelligence (AI) technology. For the forest industry, this provides an exciting and transformational opportunity. As more data comes in from systems at many points in the supply chain, forest companies can mine this information for valuable insights into their operations and use it to improve their planning processes and assumptions by learning from what is actually happening in the field. This is where predictive analytics and prescriptive modeling can work together to provide continuous improvement in forest management plans and allow forest companies to make faster, smarter and more precise decisions based on continuously updated data.
Predictive Analytics and Machine Learning
Machine learning is a branch of AI that uses algorithms and historical data to predict what is most likely to happen. Machine learning algorithms, such as linear regression and decision tree, build models to help make decisions. Since new data can be added, machine learning models can constantly update their assumptions. As data flows throughout the system, the models will continuously improve their predictions, which will lead to more accurate planning models and ultimately allow planners to make faster, smarter, and more precise decisions based on real data. Further, machine learning can provide valuable insights as to which data attributes are more impactful to performance and which are not.
Prescriptive Modeling and Optimization
Prescriptive modeling supported by optimization refers to a branch of data science and operations research focused on determining the best – or optimal – course of action to achieve a given objective. Unlike machine learning, prescriptive models evaluate all solutions in a solution space to provide the optimal path forward. Models formulated with mathematical programming methods, such as Linear Programming and Mixed Integer Programming, are frequently used in strategic, tactical, and operational forest planning to generate harvest and delivery schedules that balance revenue and volume objectives with capital and sustainability constraints. Volume assumptions for the forestland are key to these models, as are productivity assumptions for both harvest and delivery activities. As such, the accuracy of the model results is highly reliant on the accuracy of these underlying data assumptions; garbage in, garbage out, as the saying goes.
Putting it All Together: Smarter Solutions for Forestry
There is a lot to learn from historical forestry data, and AI technologies can accelerate that learning. Employing predictive machine learning and prescriptive modeling on a live operational planning system allows forest companies to leverage live data as it comes in to unlock further intelligence and continuous improvements through each planning cycle. A forest planning platform that can capture field data alongside planned values enables this continuous refinement by using the insights garnered from machine learning to update the prescriptive models’ base assumptions.
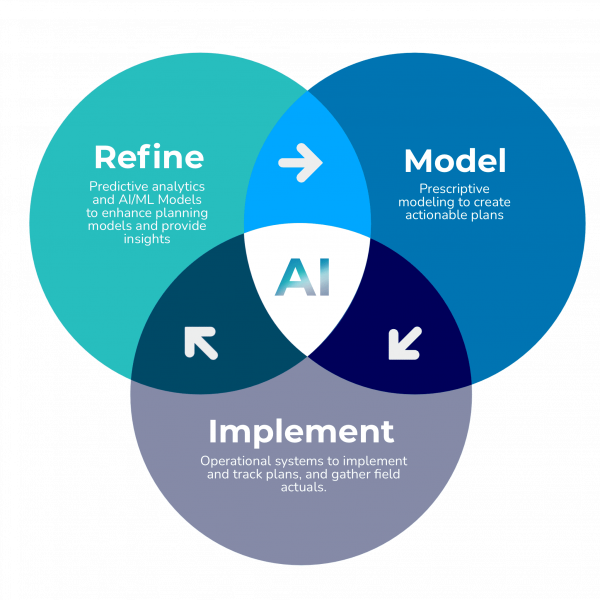
Better Outcomes with Remsoft Operations
Remsoft Operations, Remsoft’s cloud-based application to manage, visualize and measure your forest operations, leverages both predictive machine learning and prescriptive modeling in a new suite of capabilities focused on this continuous improvement cycle. These new capabilities will be initially applied to three core forestry aspects: providing more accurate assessments of productivity, better inventory estimates, and more in-depth insights.
Accurate Productivity Assessments
By analyzing the performance of harvest crews on completed units, Remsoft Operations’ new AI models will be able to calculate more accurate productivity rates for a crew’s new assignments, which, in turn, leads to more accurate end date projections. This is achieved by considering many different attributes associated with the harvest unit and the crew, including production distribution, piece size, season, and the crew’s past performance. Decision-makers and planners can view actual values from the field alongside values forecasted from AI algorithms, providing more certainty in their planning, and enabling users to detect and correct any issues. As more and more units are added to the system, the productivity models continue to learn and improve over time. And this approach can be applied to delivery productivity as well, enabling transportation planners to detect and correct deviations in the delivery plan that impact margin.
Better Inventory Estimates
Remsoft Operations’ AI models analyze unit volumes coming in from the field to determine which attributes are impacting the estimates, and then use this information to improve inventory projections for active and future harvest units. Using data such as tree species, unit profile, and treatment type, the AI model is able to predict which units will have higher volume variance. By combining revised inventory estimates with more accurate productivity assessments, the end date of a unit assignment along with potential delays can be predicted with a higher level of confidence. This enables forest planners to know where, when, and why delays may occur, and then take the appropriate actions.
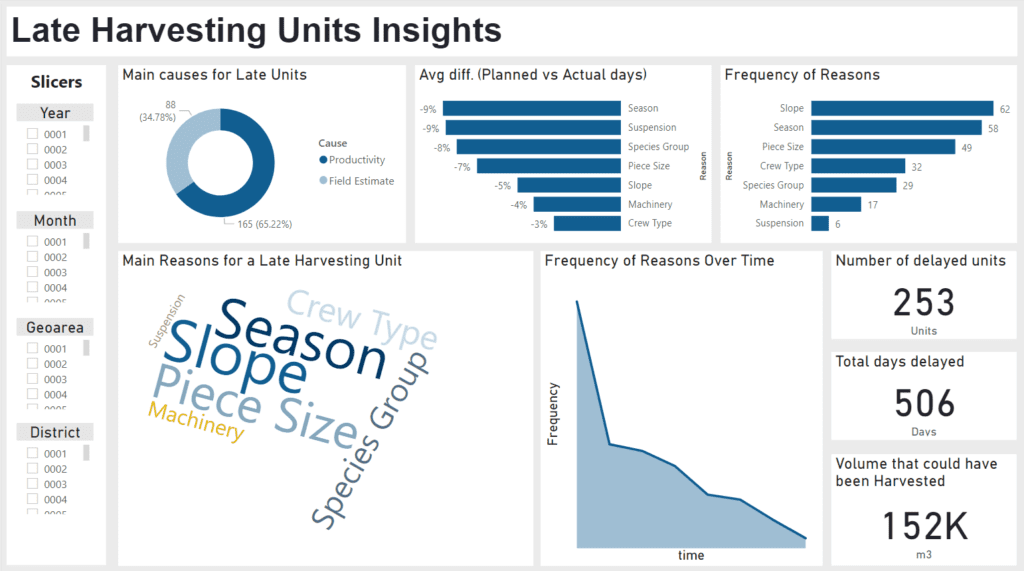
In-depth Insights
Remsoft Operations’ AI functionality provides significant insights into a forest operation. AI-based reports and analytics can highlight areas where volume estimations may be at risk, along with which unit attributes are contributing to a delay in harvest or delivery. For example, in the case of late harvesting, suspension of activity may be a relatively infrequent occurrence, but it has a substantial impact when it occurs. In contrast, slope may be a more frequent issue, but its effect on harvesting time not as damaging. This situation can be well understood in a comprehensive dashboard of insights that includes intuitive analytics, such as word clouds, ranked graphs and info cards.
The Path Forward
Helping to improve the accuracy of data flowing into a planning model, enabling better volume and productivity estimations, and providing insights into the performance of a forestry operation are some of the many areas where AI-enabled technologies can bring real value to forest companies around the world. Remsoft Operations will be adding these new capabilities to its growing feature set, helping planners create better and more defendable schedules. And the work will not stop there; stay tuned in the months to come for more AI-enabled improvements to both Remsoft Operations and our modeling suite.
LEARN MORE:
-
Find out more about Remsoft’s intelligent decision-support capabilities for forest operations planning.
-
Contact us to explore how optimization and supply chain analytics can help you solve your biggest planning challenges.